Visualizing an Ontology
Knowledge management thrives on big graphs but also requires an abstract conceptual domain-specific framework in order to regulate complexity. Developing an ontology benefits much from proper tooling to bring forward the conspicuous elements of the domain and the intricacies of the ontology. We show how yFiles with its many enticing layout algorithms can empower you and deliver visualization and interactivity no other ontology application has.
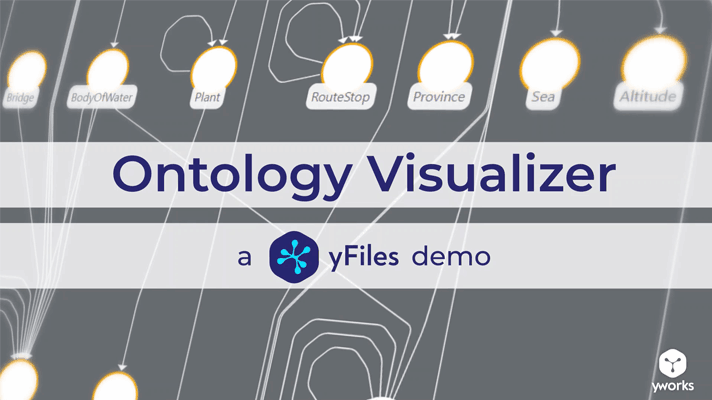
The database landscape
Relational databases come in all shapes and sizes, from the lightweight SQLite to DB2 on mainframes over Oracle 19 and MySQL. They all have in common the fact that you cannot store data without designing in advance a table structure, often called the table schema. It means defining what sort of data you wish to insert, how it’s structured, giving names (table names and fields), and how things are related to one another (foreign keys). In large enterprise applications, this process can be a whole project on its own and demands a good understanding of the business context as well as a solid comprehension of the actual database system (say, defining indexes and partitions).
The database landscape is, however, much more than relational systems. More generically, the data storage world is a jungle of possibilities and opportunities with things like key-value stores, document stores, graph databases, time-series databases, hybrid systems, cloud-only systems, and much more. Graph stores have, in particular, exploded in recent years and represent on their own a spectrum of approaches. Broadly speaking, one can divide the graph storage solutions into property graphs, triple stores, and hybrid systems. We go deeper into these three segments in another article and only sketch here the mechanics of triple stores.
Triple stores
Let’s take the simple statement 'Anna owns a Toyota.' This can graphically be represented like so
One distinguishes three parts here: an endpoint representing Anna, an endpoint representing a Toyota, and an edge with the label 'owns a.' In triple language one speaks of an SPO triple; Anna is the Subject (S), the Toyota is the Object (O), and the edge is the Predicate (P). Things couldn’t be more natural, and one can model things further in the same fashion:
In the end, this results in a stack of SPO triples which can be tabulated
-
Anna - (owns a) → Toyota
-
Anna - (works at) → yWorks
-
Anna - (uses a) → Yellow umbrella
and stored, indexed, queried as such. This, in a nutshell, is a triple store.
Note the simplicity of this scheme:
-
You don’t need to describe in advance what you wish to store. Every triple is, in essence, just an array of three strings
-
Parsing and indexing triples is fast and straightforward.
-
Storing multiple edges between endpoints is just a bunch of additional triples.
The first item deserves special attention here. It says that unlike relational systems, you don’t have to describe in advance what you wish to store. That is, one has a storage mechanism without a schema, without a predefined structure. Of course, we all are nowadays used to MongoDB and NoSQL solutions, which all are schema-less. If you have used NoSQL solutions with big data, you are for sure aware of the disadvantages of this:
-
Without structure, you end up with a heap of amorphic data.
-
The integrity of data is difficult to maintain.
-
Without a schema, different people can store the same thing in different, ambiguous ways.
What is an ontology?
When looking at triples, you can easily see it happening:
-
Somebody can say 'Anna owns a Toyota' while somebody else can state 'Anna is driving a Toyota.'
-
When updating 'yellow umbrella' to 'red umbrella,' you need to check the existence of an umbrella object first. But what is the predicate? 'uses an umbrella,' 'carrying an umbrella' …?
-
You cannot delete an endpoint because it might be used by other endpoints
and so on. There are plenty of ambiguous situations, and one cannot use triples for large-scale knowledge management without some form of schema: enter ontologies.
An ontology is a specification of a conceptualization. That is, an ontology is a description and a formal specification of concepts and their relationships. It describes part of our world, corresponding to some knowledge domain. One has ontologies for cancer research, for forensic analysis, for properties of molecules, and so on.
Looking at the example above, we convert the concrete example to something a little more abstract:
-
Person - (owns a) → Car
-
Person - (works at) → Company
-
Person - (uses an) → Umbrella
with the original example being a concrete example of these abstract relations. One calls the abstract entities, like Person, a class of the ontology. Classes are concepts, and the concrete examples of a class are called instances or individuals of the class. In our example above, Anna is an individual of the class Person.
Properties: adding data to entities
The relations emanating from the classes can be divided into two categories: things which link two classes and things which adorn a class. For example, a Person has a birthdate and a shoe size, but usually, these properties are not considered worth a class 'Birthdate' or a class 'ShoeSize.' One can also see it like this; some things around us have a complex sub-structure while others don’t. Usually, one does not assign a complex sub-structure to a number, but one does to a car. Hence, things that can be described by simple data types (number, date, string, …) are called data-type properties.
Properties that point to something more complex are called object properties. The concept 'age of a person' is a data property while 'owns a car' is an object property. The distinction is not always easily made, and this is where domain experts come in. For example, a person has an address, and this can be stored as a simple string or as a structured object (separating postal code, address-line, … into separate properties). Whether one shape is right or wrong depends on the global context and aim of the data. It’s also not uncommon to start with a data-type and months later moving on to an object. The situation is, in fact, true for any schema: it evolves together with the software and increasing complexity of the data.
Querying triple stores
How does one query triples? Triple data comes with its own query language called SPARQL. It looks similar to SQL but is tuned to the graph-like data structure. It allows one to harvest triples and subgraphs quickly. Much like SQL, every triple storage system implements the SPARQL standard and adds custom elements (query functions) to it. It is, however, pretty much the only common denominator. Aside from SPARQL, triple stores vary very much in terms of feature set, and the market is rapidly evolving. The same can be said about the associated data access frameworks wrapping around SPARQL; quality and sophistication differ a lot across the various package repositories. At the moment, Java is without a doubt your best choice if you wish to go full scale.
As a side-note, ontologies are often equated with taxonomic hierarchies, but they are not the same thing. A taxonomy is basically a naming convention, a set of labels if you wish. Usually, when these labels are organized, they form a tree-structure, and one ignores the possible non-linearity. That is, the same 'thing' can have multiple parents and form a graph rather than a tree. The reason that taxonomies are preferred over ontologies is that trees are so much easier to manipulate and represent than graphs.
Representations of ontologies
This brings us to representations of ontologies and how it relates to graph stores. From the discussion above, it should be clear that an ontology is itself a bunch of triples. This is a big contrast with the relational world where schema and data are two very different things in a database. Not so in a triple store: the schema and the data are next to another, and they are indistinguishable on the data level. Even more, you can have multiple ontologies inside a database. This can be an advantage, but it’s often the ugly aspect of triple store solutions; there isn’t a triple store that enforces the defined ontology. Read again: you can add an ontology to your triple store, but it will not be used to enforce the data to behave accordingly. Some hybrid solutions like TypeDB fix this, but most vendors do not. The implications are many, but we go deeper into this in another article. Note that because an ontology is a set of triples, you can construct and manipulate an ontology like any other triple data. Using the SPARQL query language, you can uniformly approach data and ontology within the very same queries. It allows one to dynamically alter a schema and its related data, something totally alien to the relational world.
Ontologies in the real world
The concept of ontologies can be found everywhere, e.g. DBpedia is a triple store that replicates all of the facts that can be inferred from the information stored in the whole Wikipedia. If you look at the DbPedia ontology, you discover many common-sense relations we all know. For example, a person has children, but a car does not.
You can find here an extensive list of open ontologies out of which the BioPortal is the most famous one. It contains around ten million classes related to biomedics.
From a software engineering point of view, there are not many ontology editors. The best known is Protégé from Stanford. It does a good job for small to medium-sized ontologies and is freely available.
Representing (that is, visualizing) ontologies is, however, not hard, and we have assembled one such application for you!
Creating your own ontology visualizer
yFiles is a commercial programming library designed explicitly for diagram visualization and is a perfect fit for the challenges of ontology visualization. It provides an extensive set of predefined styles for visualizing the elements of a network, while it also supports the creation of user-defined custom styles. yFiles comes with a complete set of layout algorithms for arranging the graph based on the user’s needs. These sophisticated algorithms organize the network to produce a clear and concise diagram.
Building on these powerful diagramming features, assembling an ontology visualizer with yFiles is indeed a walk in the park. The most challenging part is the data access to fetch the triples and hand them over to the yFiles framework (the GraphBuilder in the case of yFiles for HTML to be precise). This part is specific to your environment (Java, .Net, NodeJs…). Every ecosystem has its own stack of packages to approach data and triples in particular. Also, triple storage systems differ widely with respect to features, scalability, and enterprise readiness.
On the other hand, if you wish to experiment with triples and ontologies, you don’t need to crack a safe. Apache Fuseki is an easy to install cross-platform triple store, and the Protégé application mentioned above uses a simple file system. To make things easy, our yFiles for HTML-based visualizer sample application does not require any setup at all. It relies on a key-value storage system akin to what every browser is using to store information. Just run and experiment. Some of the more salient features you will find in this application are:
-
The possibility to use an excellent set of layout algorithms from yFiles. While there are various good-looking ontology visualizers out there in the open, none of them have much to offer in terms of graph layout. Usually, they rely on a simple spring-embedder algorithm that fails to deliver with large diagrams and multiple edges between nodes. No complex tuning or parameterization was necessary to achieve this, whatsoever.
-
The integrated graph analysis algorithms allowing one to gain insight into the ontological structure; using centrality measures can reveal hidden aspects of the ontology. This is a unique feature you will not find in any ontology tool.
-
Similarly, one can create clusters and box ontology classes together to define ontological sub-domains, which, in principle, can be extracted into separate ontologies.
Of course this is not where it ends. The above application is just a small sample application created within just a few days of work. However this should give you an idea already of the possibilities you get with a custom diagramming library like yFiles:
-
Use any type of data-source and technology stack to connect to your data-sources.
-
Let your users not just view, but manipulate, edit, or create your ontologies using intuitive, specific, and supportive diagram editing features.
-
Connect your application to third-party systems (e.g. to run inference engines).
-
Embed your ontology visualization into your own existing or newly created app, dashboard, or website.
So what are you waiting for? Try the sample app or start creating your own with yFiles, today!
Get the source code
The source code of the Ontology Visualizer demo application is available on GitHub. See the included readme file for usage instructions and implementation notes.
You need a copy of the yFiles for HTML diagramming library in order to run this application. You can download a free test version of yFiles in the yWorks Customer Center.